Educating autonomous networks: How China Telecom is harnessing large language models
China Telecom hopes its approach to building GenAI-based large language models (LLM) for autonomous networks will allow it to avoid the pitfalls of generative AI systems such as ChatGPT.
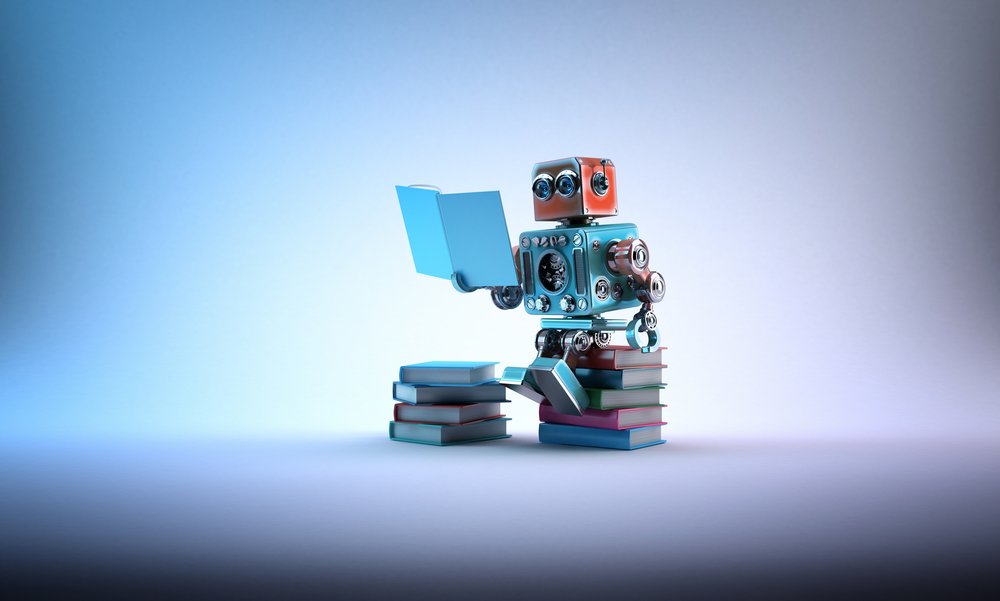
Educating autonomous networks: How China Telecom is harnessing large language models
The GenAI tool, ChatGPT, has sparked much debate about what AI-based large language models (LLM) can do. It has also exposed their limitations and risks, including a tendency to make up answers. So should LLMs be let anywhere near a telco function as business critical and dependent on reliability as autonomous network operations?
It all depends on how you harness LLM functionality, according to Qian Bing, R&D Director, China Telecom, who explains in an interview with Inform how the operator is building its own AI-based system to help analyze and address the root cause of faults in its network.
The first thing to know about China Telecom’s system is just how different it is from ChatGPT. For a start, it is not being let loose on the public internet. Instead, China Telecom is using a ChatGPT-like approach to analyzing texts and generating answers, but in association with its own knowledge graph.
“LLM can perform well in summarizing, reasoning, and content generation, but due to its probabilistic nature, it is clearly limited in scenarios that require a high accuracy of results. Combining large-scale knowledge graphs with LLM will become a future technology trend,” believes Bing, who adds that during the “rapid development of LLM technology … the interpretability feature of knowledge graph technology stands out and becomes obvious.”
Bing defines a knowledge graph as “the connection of structured and unstructured data to provide applications such as knowledge retrieval, knowledge reasoning, and intelligent questions and answers." But it is limited. As a result, when you ask the question “what happens if x fails in my network, you can only query the extracted knowledge through the knowledge graph,” explains Bing.
With the addition of an LLM, however, “you can look up and summarize more answers from the information that you failed to extract, such as the reasons why this … failed, how to determine if this … failed, etc.”, he says. “Between the knowledge graph and the ChatGPT-type system you can find an answer, but you need to be confined by expertise. You cannot be prescriptive enough.”
The fruits of knowledge
China Telecom’s research teams have spent many years refining the operator’s use of knowledge graphs to make predictions and decisions, initially as part of AIOps projects, and now as it works to make its networks autonomous.
The use of large knowledge graphs has already delivered business benefits to China Telecom, by enabling it to identify a causal relationship between separate network faults at different times that have improved the accuracy of its predictions.
As a result, “we've seen significant improvements in productivity,” explains Bing. “From the fault discovery, root cause analysis and decision making, through to executing and evaluating the whole process, the production efficiency was increased by up to 50 per cent compared to manual processes, based on tests in the real network,” he says.
The AI-based LLM system, however, goes a step further by giving “a better understanding than the knowledge graph of what happens, using theoretical studies to support its answers,” according to Bing.
China Telecom, however, is having to invest considerable resources to ensure its answers are accurate. “In AI fields people tend to say ‘rubbish in, rubbish out’. We have to check the data very carefully as this is a search engine,” says Bing. And it is an arduous, time-consuming process.
“The work of data collection is very huge, as is data labeling, most of which is manual. It needs a lot of expertise and time to extract, label and audit data,” according to Bing. “So, it needs a big investment in the early stages, while you learn it and practice it and only then can you start doing it more efficiently,” he explains. Indeed, China Telecom’s teams have “nearly three years of work in building knowledge graphs ahead of them. There are a huge number of scenarios to cover.”
Once China Telecom has established the models for collecting and labeling data, it plans to move gradually towards automating the task.
“We will study the manual processes and establish more automated processes and knowledge extraction technologies. So, the future process could be machine-based, and you only need to validate it.”
Level 4 network automation
In the meantime, network automation is gradually picking up pace. Currently, China Telecom has achieved level four automation for around 7 of 100 different wireless network operation scenarios, with “most scenarios today at level two or three” according to Bing.
However, Bing’s teams aim to add between 10 and 20 additional scenarios to the platform and move them towards level four before the end of this year, with the objective of achieving full level 4 autonomy for most of the wireless network by the end of 2025.
In addition, the operator is looking at how it could apply the output of its AN models to a digital twin. Ideally, a digital copy of a network allows operators to simulate and visualize how changes to factors such as the position and usage of network elements, customer activity and environmental conditions impact overall network performance. They could then use this understanding to optimize real life networks and improve customer experience.
Currently, China Telecom is validating its proof of concept in a TM Forum Catalyst. But creating a digital copy of a real network requires the accurate capture of multiple layers of interrelated data. And certain things lie beyond an operator’s control, such as thunderstorms or someone putting up a rooftop near a base station, for example. “There are critical aspects we are not yet able to copy to a real-time digital twin that will require a lot of research,” says Bing.
But although a digital twin is a nice-to-have, it is not essential for China Telecom to achieve its goal of level 4 network autonomy. What is pressing, however, is ensuring employees understand and support the move towards autonomous networks.
“We all agree that AI and automation technology can improve network capability, but in the actual promotion, we need to consider various factors such as the impact on the existing network, benefits [across the company], and technical feasibility," says Bing. "It is difficult to fully realize network intelligence, and we are confident that we can achieve most L4 scenarios in 2025 by developing strategies and technology, marketing and promotion, and the ... division of labor [across] teams to promote this objective.”