Combining the power of AI and APIs to automate 5G operations
The only way to deliver that end to end automation for 5G services is through AI. This proof of concept Catalyst project demonstrates the pragmatic approaches to geting there.
16 Jul 2020
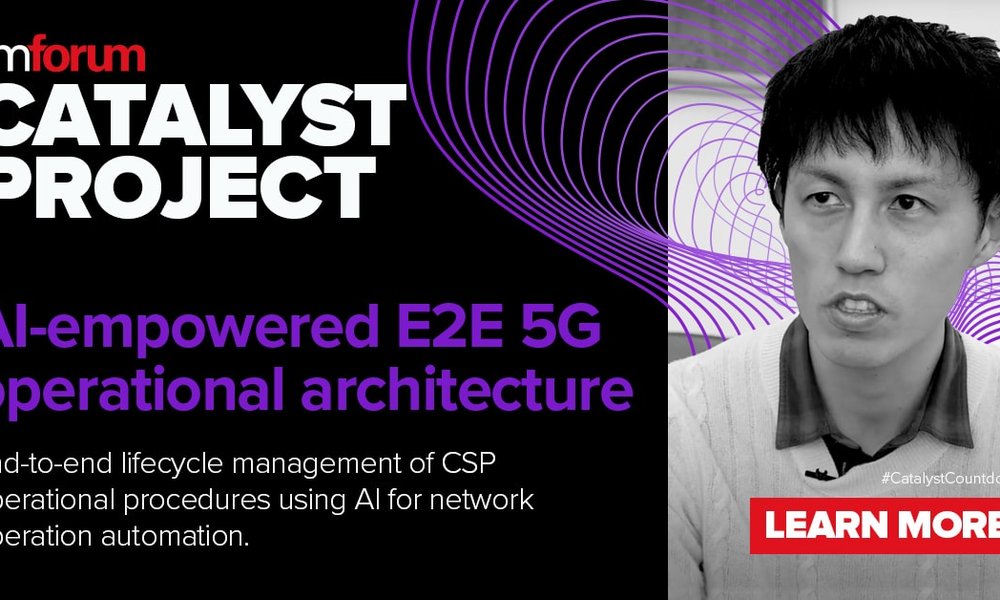
Combining the power of AI and APIs to automate 5G operations
End to end automation is indispensable for 5G enterprise services enabled by network slices. The only way to deliver that automation is through AI. This proof of concept Catalyst project demonstrates pragmatic approaches to deploying AI end to end, and points to the next steps towards improving the accuracy of AI and the automation it enables. In particular, this Catalyst underlines why 4G approaches cannot work in this 5G environment.
Automation is already deployed in some parts of communications service providers’ (CSPs) 4G networks, but operations teams rely on coding or scripts which require manual intervention. They can be written in several programming languages which adds to the complications and the process is expensive and prone to error.
This manual process cannot be scaled, nor is it fast enough for network slices where time to market needs to be under an hour. This all in an environment where new services and changes are required as close to on demand as possible meet different use cases.
In particular, it showed how to cut the cost of coding for automation. This includes: deploying AI to translate customer intent into a network requirement; designing the network infrastructure; closed-loop automation for root cause analysis and fault detection; and generating workflow to manage faults.
These different sets are necessary for end to end automation because different tasks and processes are involved at different times, in different places: For example, provisioning is not the same as maintenance.
One big lesson the team learned was about using data to train the AI. Dr. Otani says, “Everyone knows that AI is based on big data – it needs to run plenty of data to learn the behavior that is required. We studied the data generated by network operations and found out that operational data is not enough to develop good AI.
While network functions virtualization (NFV) has not been that widely deployed for 4G networks where physical network functions are deployed in the main, it is very common in 5G.
He accepts that the Catalyst does not achieve 100% accuracy with the AI, but argues that if it could get to 90%, with the other 10% addressed by humans working alongside the AI engine, this would be a game changer and he wants to make sure this message is clear to the telecom industry.
It is the goal the team is working towards, and the documentation it has provided from its work is a solid enough foundation to think about commercialization. The team quotes the reference architecture of the TM Forum Service Management Standards for AI and proposes a framework for an AI Management Standards Reference Architecture in the AI and Data Analytics Management Standards workstream. The idea behind the proposed architecture is deployed, they automatically expose their APIs to the management function.
It also hopes have input to the Forum’s Open APIs initiative because the current AI approach is a separate implementation for delivering, deploying and operating. Tatsuji Miyamoto, Associate Research Engineer at KDDI Research, says, “We need to connect them and define the data model to do that. We have started work on TMF 915, which is the AI Management API Component Suite”.
Now available on demand:
Automation is already deployed in some parts of communications service providers’ (CSPs) 4G networks, but operations teams rely on coding or scripts which require manual intervention. They can be written in several programming languages which adds to the complications and the process is expensive and prone to error.
This manual process cannot be scaled, nor is it fast enough for network slices where time to market needs to be under an hour. This all in an environment where new services and changes are required as close to on demand as possible meet different use cases.
The Catalyst, AI-empowered E2E 5G operational architecture, is championed by KDDI Research with participation from Hitachi, NEC Corporation and NICT. It demonstrated end-to-end lifecycle management of CSPs’ operational processes using AI for network operation automation at the Catalyst Digital Showcase on July 7.
In particular, it showed how to cut the cost of coding for automation. This includes: deploying AI to translate customer intent into a network requirement; designing the network infrastructure; closed-loop automation for root cause analysis and fault detection; and generating workflow to manage faults.
Dr. Tomohiro Otani, Executive Director at KDDI Research, explains, “We implemented a prototype or a capability in various processes in operational lifecycle – the planning, deployment operational and maintenance phases. We invented four different sets of AI to achieve end to end automations.”
These different sets are necessary for end to end automation because different tasks and processes are involved at different times, in different places: For example, provisioning is not the same as maintenance.
One big lesson the team learned was about using data to train the AI. Dr. Otani says, “Everyone knows that AI is based on big data – it needs to run plenty of data to learn the behavior that is required. We studied the data generated by network operations and found out that operational data is not enough to develop good AI.
“We changed our approach and used actual data for simulation in a test bed and found the AI learned faster and more precisely from the test bed than the actual network data.”
While network functions virtualization (NFV) has not been that widely deployed for 4G networks where physical network functions are deployed in the main, it is very common in 5G.
Dr. Otani explains that is it easy for the Catalyst team to, “Create the same operational environment using virtual network functions (VNFs) to generate data to develop and train AI and improve its accuracy.”
He accepts that the Catalyst does not achieve 100% accuracy with the AI, but argues that if it could get to 90%, with the other 10% addressed by humans working alongside the AI engine, this would be a game changer and he wants to make sure this message is clear to the telecom industry.
It is the goal the team is working towards, and the documentation it has provided from its work is a solid enough foundation to think about commercialization. The team quotes the reference architecture of the TM Forum Service Management Standards for AI and proposes a framework for an AI Management Standards Reference Architecture in the AI and Data Analytics Management Standards workstream. The idea behind the proposed architecture is deployed, they automatically expose their APIs to the management function.
It also hopes have input to the Forum’s Open APIs initiative because the current AI approach is a separate implementation for delivering, deploying and operating. Tatsuji Miyamoto, Associate Research Engineer at KDDI Research, says, “We need to connect them and define the data model to do that. We have started work on TMF 915, which is the AI Management API Component Suite”.
The Catalyst demonstration also refers to TM Forum’s Autonomous Networks Project architecture and Miyamoto explains, “The team wants to bring AI and APIs together to help with automation and also to contribute to the Business Process Framework (also known as eTOM) at Maturity Level 3 which is concerned with service configuration and activation, resource trouble management, resource provisioning, resource performance management and knowledge and research.
Now available on demand:
Watch the Catalyst team showcase their project
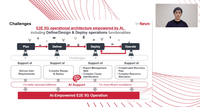