The curious case of fraud analytics
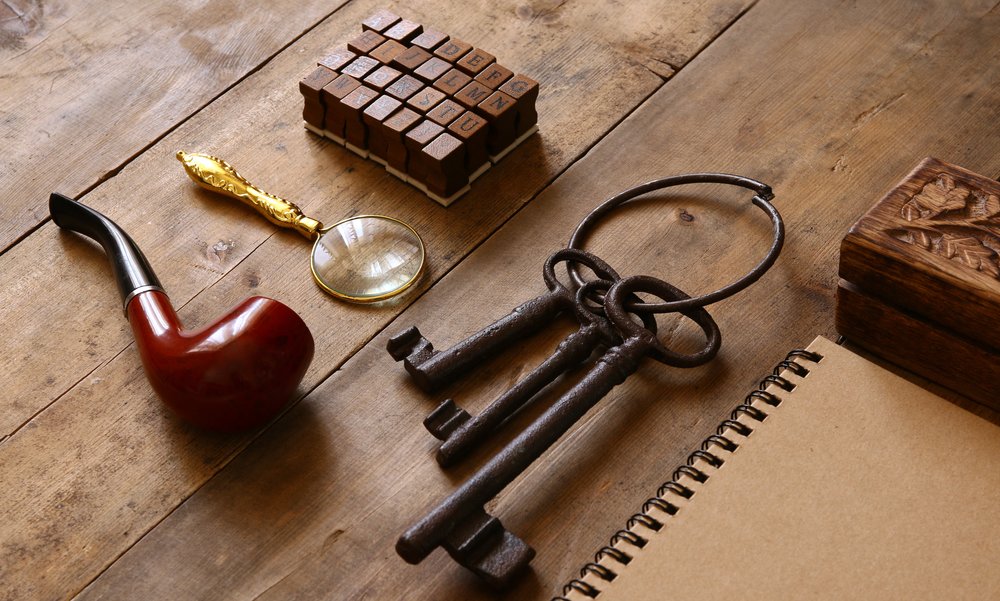
The curious case of fraud analytics
“The world is full of obvious things which nobody by any chance ever observes.” Sherlock Holmes
The same holds true in the communications service provider (CSP)/digital service provider (DSP) environment – fraud analysts must be good at spotting patterns and clues. However, a common problem is that the fraud team is often devoid of the right tools and platforms to detect any possible fraud scenarios. With millions of usage data records (UDRs) and thousands of reference data points being pushed into fraud management tools, the real and ongoing challenges faced by fraud teams are not being discussed, and this is leading to too many missed or delayed fraud scenarios. First and foremost, the role of the fraud analyst is to enable real-time fraud analysis and detection, not to spend too much time on: These tasks make the daily activities of the fraud team more complex and problematic, and can create a huge number of false positives in the systems, which are still running on traditional platforms. In order to mitigate this, there is always a dilemma around whether to apply fraud rule changes or not. Some of the most common problems in real-time detection of fraud cases in traditional databases are: The diagram below shows the various types of data feed generated by CSP applications and network platforms. It also shows a number of vulnerable areas associated with these data feeds that have been causing huge revenue losses to CSPs year after year. In order to achieve maximum fraud coverage, every single record pertaining to identified data feeds must be evaluated against all possible fraud conditions.
- Waiting for the query results
- Testing fraud management rule configuration
- Providing troubleshooting information to IT operations
- Validating/managing (uploading, deleting, adding) the reference information
- Generating manual reports (management reports, weekly reports, monthly reports, etc.)
- Manual reconciliations of fraud work (fraud loss, user performance, rule performance, etc.)
- Latency in usage record processing
- Database performance issues on longer look-back period rules
- Unavailability of visual experience
- Non-reliable reference data details (reconciliations issues)
- Manual fine-tuning of fraud engine rules
- Non-integration with dependent systems (national database, credit system, etc.)
- No learning mechanism on false positives
- Capacity issues to address new data feeds (mobile money, IoT, M2M, etc.)
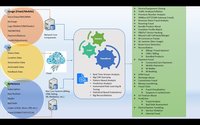
Hence, to mitigate such risks, it is of utmost importance to switch to sophisticated SQL database analytics. The challenges associated with the non-value adding activities that fraud teams have to perform on daily basis can be effectively addressed through the introduction of the technical capabilities of a big data platform, e.g.: With enhanced data spectrum availability across bands (4G/5G/LTE, etc.) there is a sharp upswing in data volume growth of various CSPs across the world. This isn’t only in the CSP space either -- with 4.7 billion global users predicted by 2025, there will also be 75 billion connected IoT devices demanding more sophisticated technology platforms for data processing, protection and security. Clearly, there is a huge need to mitigate the negative impact on revenues from ongoing and growing fraud risks. The future is in adopting big data analytics platforms and building the best analytics teams that can uncover – in real-time – the ‘hidden’ patterns being introduced through the connected world.
- Real-time processing
- Predictive analysis
- Machine learning
- Pattern-based analytics
- Data visualization support and
- Quick querying capabilities