Ooredoo Qatar tackles data models for more AI use cases
CTIO Günther Ottendorfer discusses the operator’s positive AI experiences and why he is skeptical of letting AI take full control of network operations.
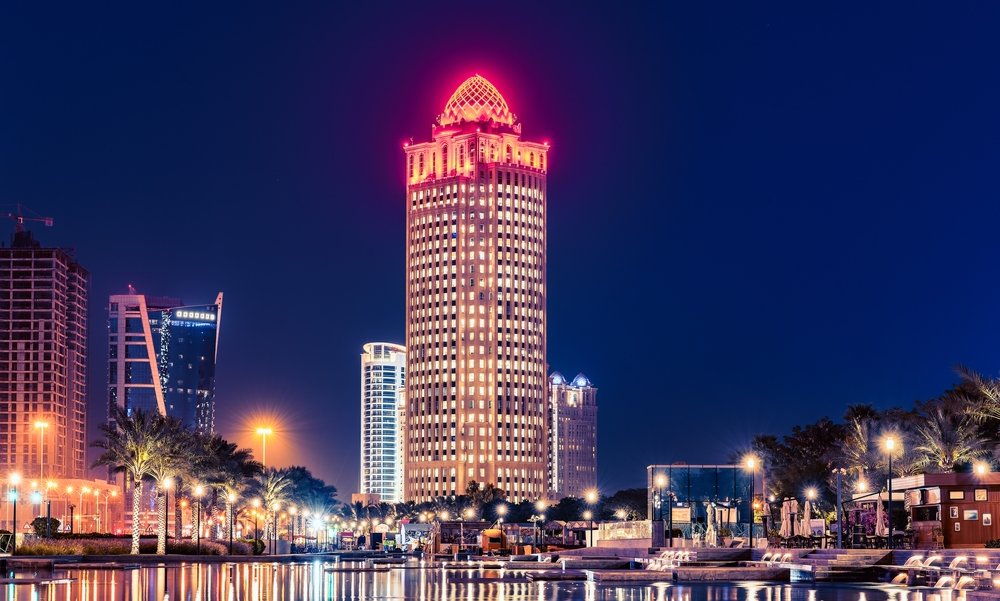
Ooredoo Qatar tackles data models for more AI use cases
Ooredoo Qatar has made strides towards its ambition to be an artificial intelligence (AI) pioneer among telcos in the Middle East. Results from its first uses of AI in operations and radio network capacity planning have been seen at some of the world’s biggest music and sports events, including the FIFA World Cup in 2022 and most recently the AFC Asian Cup.
Günther Ottendorfer, Chief Technology and Infrastructure Officer at Ooredoo Qatar, talked to TM Forum Inform about the operator’s next steps, the challenge of developing data models for new AI use cases and why fully autonomous networks are years away from reality.
Omar Daou, Head of Radio Design and Deploy, and Mohammad Abu Shawish, Director of Service Operation Center, also shared insights from Ooredoo Qatar’s AI implementations.
Ooredoo Qatar has 2.9 million fixed and mobile customers and approximately 1,200 employees. It is part of Ooredoo Group, which has 56.7 million subscribers across ten markets in the Middle East, North Africa, and Southeast Asia. The operator’s small size means it needs to be smart in the way it delivers services, and this mindset is part of what drives the AI strategy.
AI can do the “heavy lifting of going through all the data” so that people can focus on the most important things, said Ottendorfer. Also, AI enables near real-time actions, which are not possible for humans even if the operator had a bigger team.
Defining and testing data models
As the telco expands AI use cases, the focus is now on developing the data models that will best fit various sets of data from different parts of the organization. Indeed, most operators are grappling with data and building the right models for potential AI applications.
Ottendorfer said his team will work together with colleagues in the consumer and business services units to create data sets that can be used to test different AI models while also learning the best ways to test those models.
“With the data sets – be that in operations, planning, customer recommendations, or fraud detection – we can run them with hundreds of thousands of AI models to see which are suited to individual challenges, tasks, and requirements,” he said.
A key question is how much testing is needed. “Do I have to test a hundred thousand or can I focus on 100 or 10?” he asked.
Ottendorfer said he expected to have “a variety of models in our toolbox in the near future,” but the operator is “not there yet.”
Data-hungry digital twins
For the 2022 World Cup, Ooredoo worked with Ericsson and Microsoft Azure to create a digital twin of the 5G network inside the Lusail Stadium, where the final was held, to optimize capacity and detect radio interference using AI. During the tournament, people inside Lusail stadium used a whopping 222TB of data and made 2.9 million calls. At the final alone, fans used 45.1TB of data, 8.4TB of Wi-Fi, and made more than 650,000 calls.
“The software tool addressed capacity parameter tuning based on traffic flows and how people were moving [inside the stadium] … this was almost dynamic and running in an automation mode. It also helped us to identify interference and gave recommendations to adjust parameters,” said Omar Daou, Head of Radio Design and Deploy at Ooredoo Qatar.
“Such real-time actions are very helpful to improve the customer experience in stadiums,” he explained, noting that some of the functions were running in a closed loop.
Ooredoo uses the AI-powered radio capacity planning tool in hotspots like stadiums, mainly because creating a digital twin for its entire network would be a major undertaking.
“If you want to have a good digital twin, you need to fill it up with a lot of data. And that data usually you don't have. You have it in a small, controlled environment like a stadium, but not for the full real world [network]… And then the other challenge is how do you keep it up to date? That's a Google Maps challenge. It's one thing to have [data] in that one point in time, but then things change,” said Ottendorfer.
AI automated operations, up to a point
In operations, Ooredoo has reduced mean-time-to-repair (MTTR) by 30% using AI-based anomaly detection and continuous assurance solutions from startup Reailize, a division of B-Yond. It took a year to train the model using Ooredoo’s data sets and the software tools now monitor the operator’s network domains across radio access, core and transport.
The next steps are to explore where closed loop automation can be introduced, but the operator is being cautious here and limiting possible applications to “straightforward” actions that it knows are “safe,” such as basic parameter changes or a product reset.
Currently, Ooredoo uses automated anomaly detection and root cause analysis, but decisions about which actions to take are the responsibility of the operations team, explained Shawish. Next, the operator is working on automated ticketing to generate and assign trouble tickets to the team.
“For some use cases, we are calibrating the system to see how accurate it is and we may implement closed loop to further minimize MTTR,” said Shawish.
Ottendorfer said Ooredoo Qatar has done 80% of the journey towards fully autonomous networks but is sceptical that current AI models can be relied on to handle the last 20%. Like doses of medicine, he said AI needs to be administered in the right way and “too much is not healthy.”
“If you let AI run, fully autonomous, I would question where are the safeguards? How do I know that it doesn't suddenly make a mistake and deteriorate my performance? So, we are several years away from this and maybe even a decade because what you would need is an automatic quality control. Currently, the quality control is us,” he said.
As with autonomous driving, he said it is foreseeable that more automation will be added to networks slowly over time. “But remember, there were incidents with autonomous driving. As a telecom operator, you cannot afford these accidents.”
AI development takes time and collaboration
Ottendorfer said developing AI for telco use cases can be “easy” when it is for specific outcomes but not when it is part of a more general approach. For Ooredoo’s implementations in networks and operations, “we had quite a clear vision what we wanted to achieve and that’s why we were successful,” he said.
“Also, we were not in a hurry. We knew it would take some time, especially for it to become really useful, because of the iterations in these models and the time they need to digest the data sets. …And we're not that far,” he said, because the network is dynamic, and the models keep learning and adjusting with time.
Looking ahead, he expects to see operators work together on AI.
“If you take the TM Forum API approach, I think we will see a similar approach coming in AI. There is a bit of hype and smoke around it, but when it clears up, I think we will see a structured way where operators will cooperate on this,” he said.
Implementing AT will be a hot topic at DTW24 – Ignite. View the agenda: DTW24 - Agenda (tmforum.org)
Find out more about TM Forum's work on Data & AI.