Intelligent network prediction practice between Huawei and China Telecom
Data lake and AI training platforms in the cloud can be introduced to greatly reduce the AI introduction threshold and improve AI development efficiency.
12 Nov 2019
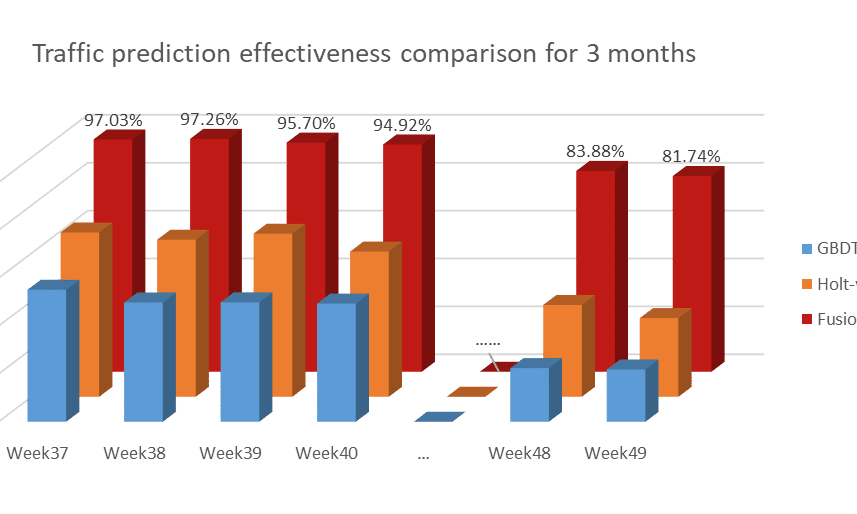
Intelligent network prediction practice between Huawei and China Telecom
At Digital Transformation Asia this week, Wesley Cheng, CTO of Huawei NAIE Domain will be discussing AI prediction practice alongside China Telecom, covering the work they've done together. Read his insights below before tomorrow's presentation.
As an infrastructure of information communication, telecommunications networks can potentially make great use of artificial intelligence (AI) technology.
A crucial industry issue is how to use the powerful analysis, judgment, and prediction capabilities provided by the AI algorithm, to empower network elements, networks, and service systems and combine them with the planning, construction, maintenance, operation, and optimization of telecom networks.
However, the introduction of AI in telecom networks faces many challenges. Useful data is difficult to acquire. Although the telecom network's data is large, the data that can be effectively used is small because of how unintelligible much of the data seems to be. Data is also missing, abnormal, and repeated and subsequently difficult to apply. Thus the development threshold for AI applications in the communications field is high, the efficiency is low, and the effect is uncontrollable. Algorithm scientists need to spend a lot of time understanding business scenarios as the AI experience in telecoms is small. There are many AI algorithms, a wide selection scope, high trial and error costs, long model training period, and poor replicability of models.
Data lake and AI training platforms in the cloud can be introduced to greatly reduce the AI introduction threshold and improve AI development efficiency; something we called the Network AI Engine (NAIE). Huawei is cooperating with China Telecom to explore the wireless network cell anomaly detection and radio cell capacity prediction scenarios based on the NAIE.
China Telecom's wireless network faces the following challenges:
The projects incorporates big data processing and AI technologies to implement data quality control, wireless cell anomaly detection, and radio cell capacity prediction. After trying some innovative AI algorithms, we see great improvement in the accuracy of abnormal cell detection and cell traffic prediction is greatly improved. Preliminary evaluations show a 12% decrease in the complaint rate of experience problems caused by capacity congestion in pilot cells, and the operations and a 20% efficiency increase in operations and maintenance (O&M).
Data quality control: The NAIE data lake helps to define data collection standards and data metadata specifications based on cell exception detection and capacity prediction scenarios, and converts multiple data sources, multiple periods, and difficult to understand low-quality data into a unified data source for easy understanding of high-quality data. Preset exception thresholds based on expert experience detect abnormal data and automatically mark exceptions in batches. Data clustering is performed on 5000+ performance indicators to identify seven types of data features, such as data periodicity, trend, and burst. This provides assurance for high quality high-precision model training.
As an infrastructure of information communication, telecommunications networks can potentially make great use of artificial intelligence (AI) technology.
A crucial industry issue is how to use the powerful analysis, judgment, and prediction capabilities provided by the AI algorithm, to empower network elements, networks, and service systems and combine them with the planning, construction, maintenance, operation, and optimization of telecom networks.
However, the introduction of AI in telecom networks faces many challenges. Useful data is difficult to acquire. Although the telecom network's data is large, the data that can be effectively used is small because of how unintelligible much of the data seems to be. Data is also missing, abnormal, and repeated and subsequently difficult to apply. Thus the development threshold for AI applications in the communications field is high, the efficiency is low, and the effect is uncontrollable. Algorithm scientists need to spend a lot of time understanding business scenarios as the AI experience in telecoms is small. There are many AI algorithms, a wide selection scope, high trial and error costs, long model training period, and poor replicability of models.
Introducing AI
Data lake and AI training platforms in the cloud can be introduced to greatly reduce the AI introduction threshold and improve AI development efficiency; something we called the Network AI Engine (NAIE). Huawei is cooperating with China Telecom to explore the wireless network cell anomaly detection and radio cell capacity prediction scenarios based on the NAIE.
China Telecom's wireless network faces the following challenges:
- Network faults and experience problems are difficult to detected in advance.
- 75% of experience problems are caused by user complaints.
- Network problems are difficult to locate and solve.
- As the network complexity increases, the efficiency of solving problems only based on expert experience is low, and O&M personnel locate problems in 90%.
- Network faults and traffic are difficult to predict, and accurate network planning and control cannot be implemented.
The projects incorporates big data processing and AI technologies to implement data quality control, wireless cell anomaly detection, and radio cell capacity prediction. After trying some innovative AI algorithms, we see great improvement in the accuracy of abnormal cell detection and cell traffic prediction is greatly improved. Preliminary evaluations show a 12% decrease in the complaint rate of experience problems caused by capacity congestion in pilot cells, and the operations and a 20% efficiency increase in operations and maintenance (O&M).
Data quality control: The NAIE data lake helps to define data collection standards and data metadata specifications based on cell exception detection and capacity prediction scenarios, and converts multiple data sources, multiple periods, and difficult to understand low-quality data into a unified data source for easy understanding of high-quality data. Preset exception thresholds based on expert experience detect abnormal data and automatically mark exceptions in batches. Data clustering is performed on 5000+ performance indicators to identify seven types of data features, such as data periodicity, trend, and burst. This provides assurance for high quality high-precision model training.
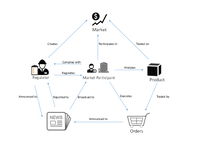
Short period capacity prediction: KD-tree is used to construct the cell space relationship which help to quickly find the neighbour cell, and use the LSTM Seq2seq algorithm to model the cell in a unified manner, so as to find out the strong traffic law caused by the user behavior. In addition, the algorithm also considers important events and weather factors to effectively predict unexpected events. For the seven-day capacity prediction and analysis, the project forecast effectiveness reaches 97%.
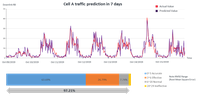
Long-term capacity prediction has no strong regularity and is affected by a large number of external factors, such as network change and tariff adjustment. The accuracy of capacity prediction is always a challenge in the industry.
By clearing the abnormal traffic fluctuation caused by non-personal factors, the project is modelled based on the cell feature clustering group, and key methods such as multi-algorithm fusion convergence, are used to improve the capacity prediction accuracy based on the data feature clustering. After analyzing the historical data of 20,000 base stations for six months, and predicting the capacity of the next three months, the fusion convergence algorithm can predict the validity of 81%, compared with the traditional Holt-winters algorithm which has a prediction validity of only 39%.
By clearing the abnormal traffic fluctuation caused by non-personal factors, the project is modelled based on the cell feature clustering group, and key methods such as multi-algorithm fusion convergence, are used to improve the capacity prediction accuracy based on the data feature clustering. After analyzing the historical data of 20,000 base stations for six months, and predicting the capacity of the next three months, the fusion convergence algorithm can predict the validity of 81%, compared with the traditional Holt-winters algorithm which has a prediction validity of only 39%.
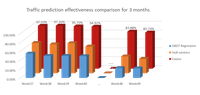
The project has achieved preliminary achievements in innovation and will continue to be incubated. In 2020, the project will be applied to China Telecom's 5G network planning, optimization, and maintenance domains. It will be managed to monitor the data quality of one million devices across the country, serving three million communications organizations and 200 million wireless network users. Our goal is to use AI intelligent anomaly detection and capacity prediction to optimize network quality before it deteriorates, and adjust traffic before any network congestion.