Are you ready to go all in on Generative AI?
Our latest research on GenAI sheds light on telcos' maturity levels across technology and domains; organizations, responsibilities and skills; data readiness and availability; governance, compliance and security; and use cases.
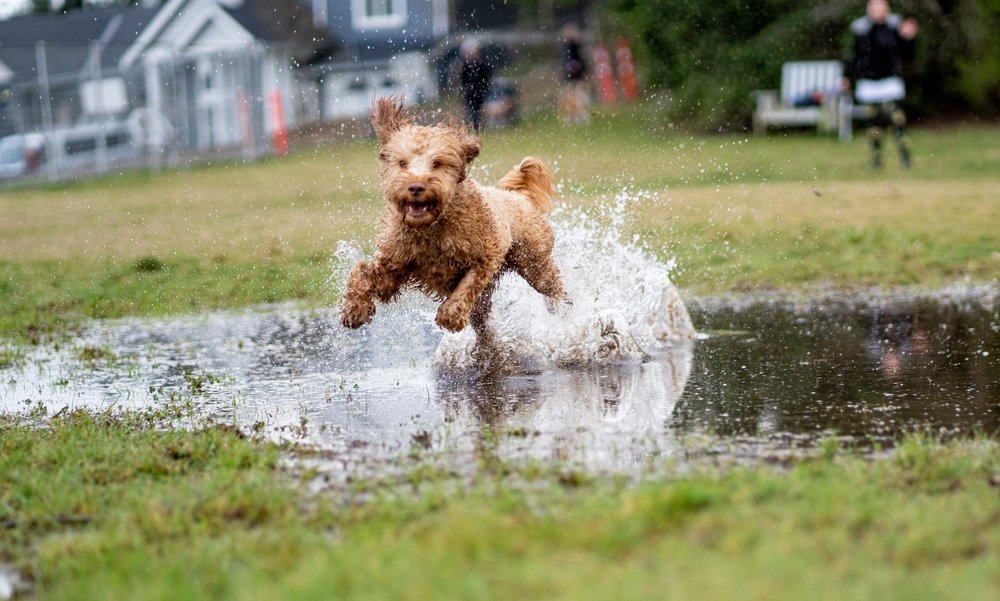
Are you ready to go all in on Generative AI?
Converting the early promise of Generative AI (GenAI) into real live use cases is proving a challenge for many CSPs. But the good news is that there are a number of operators across the world that are delivering on GenAI. They have acquired the tools to master different LLMs and to use different techniques to improve their accuracy and efficacy. They have already put use cases into production both to drive productivity and efficiency gains and to add value to the business. Transforming customer operations and the customer experience represents an immediate opportunity that can be grasped by any CSP that takes the necessary steps to build their internal competence and capabilities.
TM Forum’s Generative AI Maturity Interactive Tool (GAMIT) gives you the opportunity to compare yourselves with GenAI market leaders. Built in partnership with AWS, and on data from 203 AI decision-makers at 124 telecoms operators in 61 countries, GAMIT measures your GenAI maturity in six domains:
- Technology maturity and understanding
- Organization, responsibilities and skills
- Data readiness and availability
- Governance, privacy, compliance and security
- Business objectives
- Taking AI use cases into production
By scoring your own organization in each of these domains, or as we call them, “pillars” you can get a good sense of the areas of your business where you need to make changes or improvements if you want to start deploying AI at scale. To date we have identified:
Technology maturity and understanding
GenAI is a new technology and CSPs have had to move fast to acquire the skills to understand how to choose the best LLM and the best training, tuning, RAG and prompt approaches for each use case. Indeed, the rapid proliferation of foundational models, and the emergence of small language models as a complement to large language models, means that any teams and capabilities that a CSP assembles need to be regularly refreshed.
It should therefore come as no surprise that 71% of our respondents to date recognize they are at the relatively early stages of building their capabilities to generate the most accurate results possible for each use case, with 52% of respondents acknowledging that the same goes for assessing the cost and ROI for different use cases. Meanwhile only 22% of respondents reckon their organizations have built significant capabilities in finding approaches and techniques that provide the highest possible levels of accuracy, while 37% say they have significant capabilities to assess cost and ROI.
More broadly speaking, CSPs need to master a whole range of skills from FinOps to SecOps and MLOps to build a GenAI production line and ensure that the cost of deploying AI use cases does not undermine its success. Those operators which have already put some of these capabilities in place for their public cloud deployments have a big advantage over those CSPs which have been slow to embrace cloud computing or who have bad experiences and are now retrenching.
Organization, responsibilities and skills
However much excitement there may be about the potential of Generative AI, its adoption and deployment will not be prioritized unless there are board-level accountabilities and expert teams sitting both in the center of the organization and in the divisions and functions which will benefit from it.
Only 37% of CSPs that have used GAMIT or participated in our survey have a CXO in place who is predominantly focused on AI strategy and deployment. These roles can be Chief Data Officers, Chief Digital Officers or Chief AI officers. However, a majority (57%) have AI centers of excellence and 44% have dedicated AI roles and responsibilities in different teams and functions.
Getting the right balance between “build” and “buy” is important for all CSPs if they want to avoid vendor lock-in and minimize the technical debt that can result from deploying closed systems: 18% of CSP respondents say that they are largely reliant on systems integrators while 29% say they have recruited a large number of AI skills in teams across the organization.
Data readiness and availability
Getting fast access to quality data is essential for the successful use of any type of AI. Our respondents, for the most part, expressed a reasonable level of satisfaction with the data strategies of their organization. 68% of respondents scored themselves a 3 or a 4 out of five in terms of getting rapid access to data with 76% of CSPs also scoring a 3 or a 4 for access to trustworthy data. Where Generative AI differs from other types of AI is in its suitability for use on unstructured data. CSPs scored themselves worse in terms of getting access to unstructured data than to structured data with 72% of respondents giving themselves a score of three or less out of five.
Governance, privacy, compliance and security
Careless or irresponsible AI deployments can cause huge reputational damage to an organization like a telecoms operator. Most operators have some sort of data governance in place, which they are now upgrading to AI governance programs. But the speed of this upgrade and the capabilities of difference governance platforms varies hugely. Just over half of our respondents said that their governance program covered issues relating to security, compliance and cost. Similarly, half of responents said their GenAI governance include a well-defined process and policy that reviewed LLMs and use cases and addressed any potential negative impacts.
Business objectives
In early GenAI use case deployments there has been a strong focus on customer service operations. More specifically, CSPs have been using GenAI for summarization and document creation to make contact center teams more proactive and informed and for delivering an improved chatbot experience. But GenAI market leaders are deploying GenAI use cases for a wider selection of use cases delivering both efficiency gains and value creation. And they are using a whole range of outputs, from summarization to document, content and code creation.
More than half of CSPs (53%) say they ae putting GenAI-enhanced chatbots into production. The figures are somewhat lower for other formats with 44% putting use cases based on summarization into production and 31% using document and code creation for use cases going into production.
The most GenAI-advanced CSPs are deploying use cases across many different departments and functions in their organizations including networks, internal IT sales and marketing and their product organizations. Almost half of our respondents said that efficiency gains were the main driver for use cases in network operations (45%) and IT (43%) with considerably less (30% in IT and 29% in network operations) stating that business objectives were evenly split between efficiency gains and value creation. The picture is somewhat different in sales and marketing with 69% of respondents recognizing that value creation is either the main driver or that business objectives are evenly divided between efficiency and value creation.
Use cases
The number of use cases that a CSP has put into production provides a good indication of their level of GenAI maturity. However, it is not, in itself an indicator of maturity. Indeed, looking at AI more broadly many CSPs admit privately that use cases have not always achieved the desired ROI. This is why GenAI technology and MLOps maturity are so important. Looking across all responses to our survey and tool, the average number of GenAI use cases that a CSP has put into production is between three and six. At the extremes, 17% of CSPs have no put any GenAI use cases into production while 14% have put more than 10 into production.